Leveraging machine learning to find security vulnerabilities
A behind-the-scenes peek into the machine learning framework powering new code scanning security alerts.
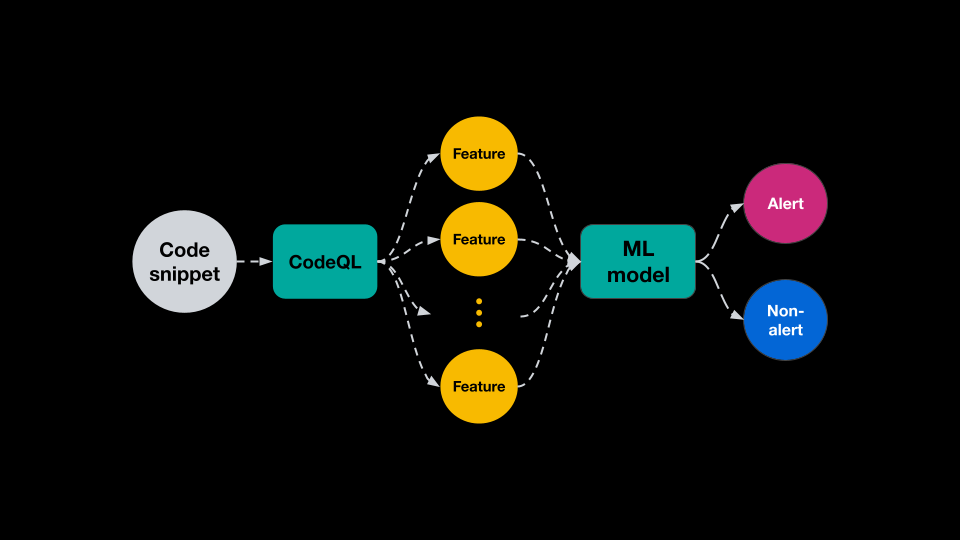
GitHub code scanning now uses machine learning (ML) to alert developers to potential security vulnerabilities in their code.
If you want to set up your repositories to surface more alerts using our new ML technology, get started here. Read on for a behind-the-scenes peek into the ML framework powering this new technology!
Detecting vulnerable code
Code security vulnerabilities can allow malicious actors to manipulate software into behaving in unintended and harmful ways. The best way to prevent such attacks is to detect and fix vulnerable code before it can be exploited. GitHub’s code scanning capabilities leverage the CodeQL analysis engine to find security vulnerabilities in source code and surface alerts in pull requests – before the vulnerable code gets merged and released.
To detect vulnerabilities in a repository, the CodeQL engine first builds a database that encodes a special relational representation of the code. On that database we can then execute a series of CodeQL queries, each of which is designed to find a particular type of security problem.
Many vulnerabilities are caused by a single repeating pattern: untrusted user data is not sanitized and is subsequently accidentally used in an unsafe way. For example, SQL injection is caused by using untrusted user data in a SQL query, and cross-site scripting occurs as a result of untrusted user data being written to a web page. To detect situations in which unsafe user data ends up in a dangerous place, CodeQL queries encapsulate knowledge of a large number of potential sources of user data (for example, web frameworks), as well as potentially risky sinks (such as libraries for executing SQL queries). Members of the security community, alongside security experts at GitHub, continually expand and improve these queries to model additional common libraries and known patterns. Manual modeling, however, can be time-consuming, and there will always be a long tail of less-common libraries and private code that we won’t be able to model manually. This is where machine learning comes in.
We use examples surfaced by the manual models to train deep learning neural networks that can determine whether a code snippet comprises a potentially risky sink.
As a result, we can uncover security vulnerabilities even when they arise from the use of a library we have never seen before. For example, we can detect SQL injection vulnerabilities in the context of lesser-known or closed-source database abstraction libraries.
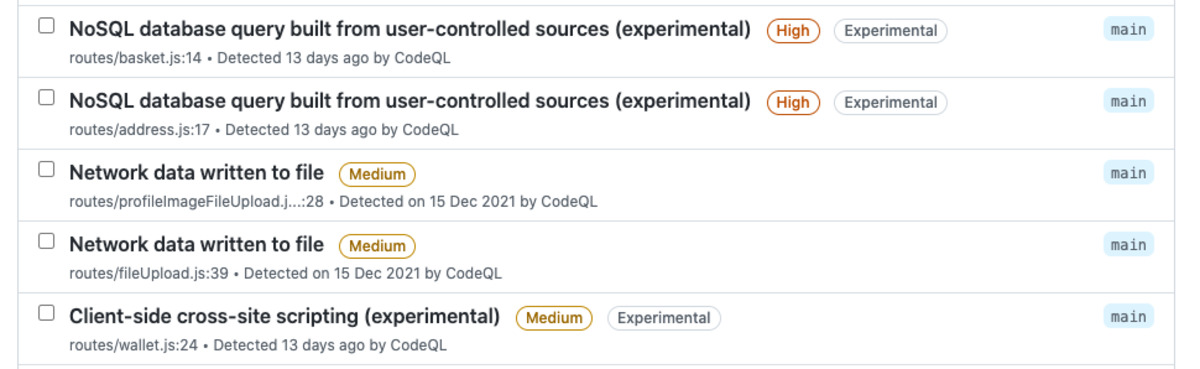
Building a training set
We need to train ML models to recognize vulnerable code. While we have experimented some with unsupervised learning, unsurprisingly we found that supervised learning works better. But it comes at a cost! Asking code security experts to manually label millions of code snippets as safe or vulnerable is clearly untenable. So where do we get the data?
The manually written CodeQL queries already embody the expertise of the many security experts who wrote and refined them. We leverage these manual queries as ground-truth oracles, to label examples we then use to train our models. Each sink detected by such a query serves as a positive example in the training set. Since the vast majority of code snippets do not contain vulnerabilities, snippets not detected by the manual models can be regarded as negative examples. We make up for the inherent noise in this inferred labeling with volume. We extract tens of millions of snippets from over a hundred thousand public repositories, run the CodeQL queries on them, and label each as a positive or negative example for each query. This becomes the training set for a machine learning model that can classify code snippets as vulnerable or not.
Of course, we don’t want to train a model that will simply reproduce the manual modeling; we want to train a model that will predict new vulnerabilities that weren’t captured by manual modeling. In effect, we want the ML algorithm to improve on the current version of the manual query in much the same way that the current version improves on older, less-comprehensive versions. To see if we can do this, we actually construct all our training data from an older version of the query that detects fewer vulnerabilities. We then apply the trained model to new repositories it wasn’t trained on. We measure how well we recover the alerts detected by the latest manual query but missed by the older version of the query. This allows us to simulate the ability of a model trained with the current version of the query to recover alerts missed by this current manual model.
Features and modeling
Given a large training set of code snippets labeled as positive or negative examples for each query, we extract features for each snippet and train a deep learning model to classify new examples.
Rather than treating each code snippet simply as a string of words or characters and applying standard natural language processing (NLP) techniques naively to classify these strings, we leverage the power of CodeQL to access a wealth of information about the underlying source code. We use this information to produce a rich set of highly informative features for each code snippet.
One of the main advantages of deep learning models is their ability to combine information from a large set of features to create higher-level features and discover patterns that aren’t obvious to humans. In partnership with security and programming-language experts at GitHub, we use CodeQL to extract the information an expert might examine to inform a decision, such as the entire enclosing function body for a snippet that sits within a function, or the access path and API name. We don’t have to limit ourselves to features a human would find informative, however. We can include features whose usefulness is unknown, or features that can be useful in some instances but not all, such as the argument index for a code snippet that’s an argument to a function. Such features may contain patterns that aren’t apparent to humans, but that the neural network can detect. We therefore let the machine learning model decide whether or how to use all these features, and how to combine them to make the best decision for each snippet.
Once we’ve extracted a rich set of potentially interesting features for each example, we tokenize and sub-tokenize them as is commonly done in NLP applications, with some modifications to capture characteristics specific to code syntax. We generate a vocabulary from the training data and feed lists of indices into the vocabulary into a fairly simple deep learning classifier, with a few layers of feature-by-feature processing followed by concatenation across features and a few layers of combined processing. The output is the probability that the current sample is a vulnerability for each query type.
Due to the scale of our offline data labeling, feature extraction, and training pipelines, we leverage cloud compute, including GPUs for model training. At inference time, however, no GPU is needed.
Inference on a repository
Once we have our trained machine learning model, we use it to classify new code snippets and detect likely vulnerabilities for each query. When ML-generated alerts are enabled by repository owners, CodeQL computes the source code features for the code snippets in that codebase and feeds them into the classifier model. The framework gets back the probability that a given code snippet represents a vulnerability, and uses this probability to surface likely new alerts.
The full process runs on the same standard GitHub Action runners that are used by code scanning more generally, and it’s transparent to the user other than some increased runtime on large repositories. When the code scanning is complete, users can see the ML-generated alerts along with the alerts surfaced by the manual queries, with the “Experimental” label allowing them to filter ML-generated alerts in or out.
Does it work?
When evaluating ML-generated alerts, we consider only new alerts that were not flagged by the manual queries. True positives are the correct alerts that were missed by the manual queries; false positives are the incorrect new alerts generated by the ML model.
To measure metrics at scale, we use the experimental setup described above, in which the labels in the training set are determined using an older version of each manual query. We then test the model on repositories that were not included in the training set, and we measure its ability to recover the alerts detected by the current manual query but missed by the older one. Our metrics vary by query, but on average we measure a recall of approximately 80% with a precision of approximately 60%.
We’re currently extending ML-generated alerts to more JavaScript and Typescript security queries, as well as working to improve both their performance and their runtime. Our future plans include expansion to more programming languages, as well as generalizations that will allow us to capture even more vulnerabilities.
Run the “Experimental” queries if you want to uncover more potential security vulnerabilities in your codebase. The more the community engages with our alerts and provides feedback, the better we can make our algorithms, so please consider giving them a try!
Tags:
Written by
Related posts
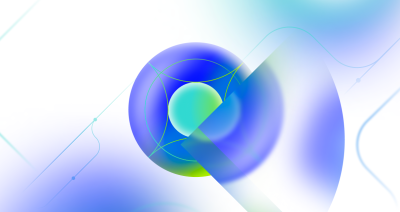
Attacking browser extensions
Learn about browser extension security and secure your extensions with the help of CodeQL.
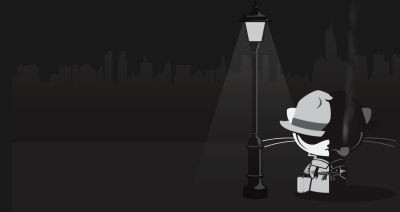
Cybersecurity spotlight on bug bounty researcher @adrianoapj
As we wrap up Cybersecurity Awareness Month, the GitHub Bug Bounty team is excited to feature another spotlight on a talented security researcher who participates in the GitHub Security Bug Bounty Program—@adrianoapj!
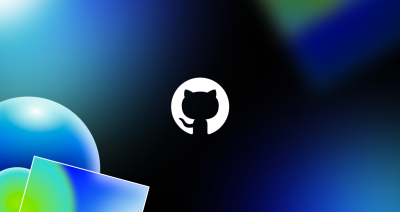
Securing the open source supply chain: The essential role of CVEs
Vulnerability data has grown in volume and complexity over the past decade, but open source and programs like the Github Security Lab have helped supply chain security keep pace.