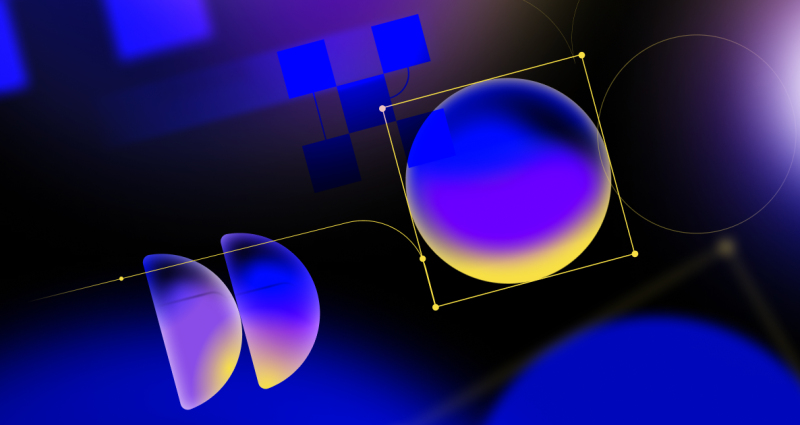
GitHub Availability Report: March 2024
In March, we experienced two incidents that resulted in degraded performance across GitHub services.
Learn how we’re experimenting with generative AI models to extend GitHub Copilot across the developer lifecycle.
Earlier this year, it seemed like every headline or dinner conversation was earmarked by the buzzwords “generative AI.” And while 2023 has been a benchmark year for the adoption of generative AI, it’s not entirely a new technology. Arguably, AI has been around since the ‘60s, but the AI as we know it today came to be with the invention of machine learning frameworks known as neural networks (you can read more about that here).
For the past few years at GitHub, we’ve been experimenting with generative AI models to create new, meaningful tools for developers—which is how GitHub Copilot was born. And since GitHub Copilot’s initial preview release in 2021, we’ve been thinking a lot about how generative AI can (and should) empower developers to be more productive at every stage of the software development lifecycle. That led us to our vision for the future of AI-powered software development with GitHub Copilot, which we covered in detail this year at GitHub Universe 2023.
In this blog post, we’ll explore some of the experiments we’ve conducted with generative AI models over the past few years, as well as take a behind-the-scenes look at some of our key learnings. We’ll also explore what going from a concept to a product looks like with a radically new technology.
As developers increasingly use AI tools to improve overall productivity, we have four key pillars at GitHub that are guiding our work and how we experiment with AI. We want a developer’s AI experience to be:
Now that we have a baseline understanding of how we prioritize experimenting with AI, let’s take a look at the events that led to the conception of the latest evolution of GitHub Copilot.
Last year, researchers from GitHub Next, our R&D department focused on the future of software development, were given advanced access to OpenAI’s large language model (LLM) that would soon be released as GPT-4.
“At the time, no one had seen anything like this,” Idan Gazit, senior director of research for GitHub Next recalls. “It became a race to discover what the new models are capable of doing and what kinds of applications are possible tomorrow that were impossible yesterday.”
So, the GitHub Next team did what they do best: experiment. Over the course of several months, researchers from GitHub Next used the GPT-4 model to develop potential new tools and features that could be used across the GitHub platform. Once the team identified the projects that showed true value, the sprint to build began.
“In classic GitHub Next fashion, we sat down and spiked a bunch of ideas and saw what looked promising or exciting to us,” Gazit explains. “And then we doubled down on the things that we believed would bear fruit.”
In the time between receiving the model and the slated announcement of the model’s release in March 2023, the team had come up with several concepts and technical previews.
At the time, no one had seen anything like this. It became a race to discover what the new models are capable of doing and what kinds of applications are possible tomorrow that were impossible yesterday.
As these projects came together, senior leadership at GitHub began to think about what these meant for the future of GitHub Copilot. Mario Rodriguez, VP of product management, says, “We knew we wanted to make an announcement of our own around the joint Microsoft and OpenAI announcement of GPT-4. At that time, GitHub Next had a set of investments that they were making that they thought were worthwhile for the announcement. Those investments were not production-ready—they were more future-focused.” He explains, “But that got us thinking, so we put pen to paper and came up with the ambition behind the latest evolution of GitHub Copilot.”
As teams at GitHub thought about evolving GitHub Copilot beyond a pair programmer in the IDE, they imagined a future where GitHub Copilot was:
This thought exercise, in conjunction with GitHub Next’s work to conceptualize and create new tools that could revolutionize the developer workflow, crystallized what would make up the latest evolution of GitHub Copilot. And on March 22, 2023, the technical preview for what GitHub Copilot would evolve into was released to the world with GitHub Copilot Chat and the following technical previews created by GitHub Next:
So, what happened behind the scenes to come up with these previews? Let’s find out.
If you asked just about any developer what’s something that is specifically unique to GitHub, it would be pretty shocking if they didn’t say “pull requests.” Pull requests play a central role in the GitHub developer experience—they’re not only a point of collaboration, but a gateway for teams to view and approve any changes to code.
So when Andrew Rice, Don Syme, Devon Rifkin, Matt Rothenberg, Max Schaefer, Albert Ziegler, and Aqeel Siddiqui were given the GPT-4 model, they were tasked with the challenge of finding ways to incorporate AI into GitHub.com.
“GitHub invented pull requests, so we started thinking, how could we add AI smarts around pull requests?” Rice says. “We tried a bunch of stuff—we prototyped automatic code suggestions for reviews, we had a sort of summarize mode, and a bunch of other things around test generation.” But as the deadline of March 22 approached, a few of these prototyped features weren’t working as desired, so Rice and team began focusing their attention and efforts solely on the summary feature.
With the early version of Copilot for Pull Requests, a developer could submit their pull request and the AI model would generate a description and walkthrough of the code in the first comment to provide important context for the reviewer.
“We did an internal study of the feature with Hubbers and it didn’t go well,” Rice laughs. It wasn’t that the developers didn’t like what the feature was trying to achieve, it was the user experience, Rice believes, they were having challenges with. “The developers were concerned that the AI would be wrong. But there’s two things: you have the content the AI generates and then you have the way that it’s presented to the user and how it interacts with the workflow. At first, we focused a lot on the first bit, the AI-generated content, but it turned out that the second bit was far more crucial in getting this thing to fly,” he explains.
To work around this, Rice and team decided to pivot and use the same AI-generated content but frame it differently. “Instead of a comment, we put it as a suggestion to the developer that let them get a preview of what the description of their pull request could look like that they could then edit,” Rice says. “So, we moved it to a suggestion system, and all of a sudden the feedback changed to ‘wow, these are helpful suggestions.’ The content was exactly the same as before, it was just presented differently.”
For Rice, the key takeaway during this process was the importance of how the AI output is presented to the developer, rather than the total accuracy of the suggestion. That doesn’t mean that it’s acceptable for the AI to be completely wrong, but it does mean that a developer’s demand for the quality of the suggestion sits on a spectrum—developers will view something as it fits within their workflow regardless of what is served to them. When the content was served as a suggestion that the developer had the authority to accept and edit, the typical attitude toward the feature changed.
Eddie Aftandilian, a principal researcher that headed up the development of another GitHub Copilot feature, shared some similar sentiments and takeaways throughout the process of building Copilot for Docs. In late 2022, Aftandilian and Johan Rosenkilde were examining embeddings and retrievals, and they prototyped a vector database for a different GitHub Copilot experiment. “This got us thinking, what if we could use this for retrievals of things other than just code,” Aftandilian remembers. “Once we got access to GPT-4, we realized we could use the retrieval engine to search a large corpus of documentation, and then compose those search results into a prompt that elicits better, more topical answers based on the documentation,” he explains.
“Since GitHub is all about developer tools, we thought, how can we make this into a useful developer tool?” Aftandilian says. Developers spend an enormous amount of time poring over docs to find solutions—and as Aftandilian plainly puts it, “No one really likes reading documentation!” He continues, “It also can be hard to get the right answer out of docs, too. So, it seemed like there was an opportunity here for something that could answer a developer’s question more directly and unblock them. It’s also an area of the development process that we felt was underexplored. We spend a lot of time searching around for answers, which can be a real pain point, and we thought we could do better with these new LLMs.”
Aftandilian, along with Devon Rifkin, Jake Donham, and Amelia Wattenberger, also deployed their early version of Copilot for Docs to Hubbers, extending GitHub Copilot’s reach to GitHub’s internal docs in addition to public documentation. But once the preview reached public testing, he got some interesting feedback about the quality of the AI outputs.
“One challenge we came across during the development process was that the models don’t always give the right answer or the right document,” Aftandilian says. “To address this, we built in the capability for our answers to provide references or links to other documentation. We found that when we deployed it, the feedback we received was that developers didn’t mind if the output wasn’t always perfectly correct if the linked references made it easier to evaluate what the AI produced. They were using Copilot for Docs as a search engine,” he says.
The UX needs to be tolerant of AI's mistakes—you can't assume that the AI will always be right.
Another key learning for Aftandilian was that human feedback is the true gold standard for developing AI-based tools. “One of our conclusions was that you should ship something sooner rather than later to get real, human feedback to drive improvements,” he says.
And similar to Rice’s earlier point, user experience is also critical to the success of these AI-powered tools. “The UX needs to be tolerant of AI’s mistakes—you can’t assume that the AI will always be right,” Aftandilian says. “Initially we were focused on getting everything right, but we soon learned that the chat-like modality of Copilot for Docs makes the answers feel less authoritative and folks are more tolerant of the responses when they point the user in the right direction. The AI isn’t always perfect, but it’s a great start.”
In October 2022, the entire GitHub Next team met up in Oxford, England to get together and discuss all of the projects that they were currently working on, as well as some exciting—and maybe even far-fetched—ideas.
“One of the things that I pitched at this crazy ideas session was a project that would use LLMs to help you figure out CLI commands,” Johan Rosenkilde, a principal researcher for GitHub Next, recalls. “I was thinking about something that could use natural language prompts to describe what you wanted to do in the command line, then some sort of GUI or interface pops up that helps you narrow down what you want to do.”
As Rosenkilde talked through his pitch, one of his colleagues, Matt Rothenberg, began writing an application that did almost exactly that. “By the time my talk ended, he asked if he could show me something, and my mind was just blown,” Rosenkilde laughs. That thirty-minute prototype was the genesis for what would become Copilot for CLI.
“What he had created clearly showed that there was something of value here, but it lacked maturity of course,” Rosenkilde says. “And so what we did was carve out time to refine this rough demo into something that we could deliver to developers,” he says. By the time March 2023 rolled around, they had a preview that brought the power of GitHub Copilot right to the CLI for developers to quickly ask for and receive their desired shell commands, including a breakdown that explains each part of the command—without ever needing to search the web for answers.
When reflecting on the process of taking this app from that original, scrappy version to a technical preview, Rosenkilde echoes Rice and Aftandilian in his appreciation for the subtlety of UX decisions.
“I’m a backend person: I’m heavy on theory and I like really difficult problems that cause me to think for weeks about a solution,” Rosenkilde says. “Matt was the UX guy, and he iterated extremely quickly through a lot of options. So much of the success of this application hinged on the UX, and that’s a lesson that I’ve taken with me. All that we do in GitHub Next, in the end, is think up tools that will add value to the user experience, so it’s crucial that we get the design right and that it fits in with what the AI model can do. As we know, the AI models aren’t perfect, but when they are imperfect, the cost to the user should be as low as possible,” Rosenkilde says.
That simple fact is what informs the explanation field that can be found in Copilot for CLI. “This actually wasn’t part of the original UI. As the product matured, we came up with the explanation field, but we had some difficulty with the LLM producing the structured type of explanations we sought. It’s very unnatural for a language model to produce something that looks like this, I had to hit it with a very large hammer,” he jokes. “We wanted it to be clearly structured, but if you just ask the AI to explain a shell command, it would feed you a long paragraph that is not readily scannable and might not include the details you want.”
Rosenkilde also felt that it was important to add the explanation field to help developers learn about shell scripts and double check that they have received the correct command. “It’s also a security feature because you can read in natural language whether the command will change files you didn’t expect to change,” he explains. This multifaceted explanation field is not only useful, it’s a testament to the UX of the application. “When you have such a small application, you want every feature to have multiple different uses so that you can package up a lot of complexity in something that visually is very simple.”
We’re focused on something great here: creating delightful AI experiences for everyone who interacts with the GitHub platform. And while we’re working on it, we invite you to be part of the process. You can get involved by joining the waitlists for our current previews and giving us your honest feedback on what you think and what you want to see going forward.
And if you’re not already using GitHub Copilot, give it a try with a free, 30-day trial for individual developers.